附件
引用本文: | 吴志明,李建超,王睿,时蕾,苗松,吕恒,李云梅.基于随机森林的内陆湖泊水体有色可溶性有机物(CDOM)浓度遥感估算.湖泊科学,2018,30(4):979-991. DOI:10.18307/2018.0411 |
| WU Zhiming,LI Jianchao,WANG Rui,SHI Lei,MIAO Song,LV Heng,LI Yunmei.Estimation of CDOM concentration in inland lake based on random forest using Sentinel-3A OLCI. J. Lake Sci.2018,30(4):979-991. DOI:10.18307/2018.0411 |
|
|
|
本文已被:浏览 12362次 下载 4980次 |
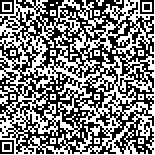 码上扫一扫! |
|
基于随机森林的内陆湖泊水体有色可溶性有机物(CDOM)浓度遥感估算 |
吴志明1, 李建超1, 王睿1, 时蕾1, 苗松1, 吕恒1,2, 李云梅1,2
|
1.南京师范大学虚拟地理环境教育部重点实验室, 南京 210023;2.江苏省地理信息资源开发与利用协同创新中心, 南京 210023
|
|
摘要: |
水体中的有色可溶性有机物(CDOM)是湖泊生态系统中氮、磷等有机营养物质的重要来源,利用卫星遥感数据反演内陆水体中CDOM浓度一直是个挑战.因此本文基于滇池2009年9月、2017年4月以及太湖2016年7月的现场原位观测和室内实验,在分析水体固有光学特性的基础上,引入机器学习算法,建立了基于哨兵-3A OLCI传感器的我国内陆湖泊水体CDOM浓度随机森林反演模型.利用独立的验证数据集对所构建的随机森林模型及常用的波段比值模型、一阶微分模型、半分析模型、BP神经网络模型等的反演精度进行评价.结果表明:随机森林模型的均方根误差为0.14 m-1,平均相对误差为21%,与反演效果相对较好的BP神经网络模型相比,均方根误差降低了50%,平均相对误差降低了38%,反演精度得到了显著的提高.根据随机森林算法的特征重要性参数提供的各自变量影响力结果,发现B11(709 nm)和B6(560 nm)波段贡献率最大,是反演CDOM的敏感波段.最后将随机森林模型应用到滇池2017年4月12日、太湖2017年5月18日的哨兵-3A OLCI影像上,得到滇池、太湖水体CDOM浓度分布图.滇池CDOM浓度的分布特征大致符合东北、西南高,中西部低的趋势,且河口处的CDOM浓度高于湖泊水体,表明径流的输入给滇池水体带来了大量的CDOM.太湖CDOM浓度的分布特征大致符合西部高,湖心区和东部低的趋势.太湖西部以及北部梅梁湾受入湖河流影响较大,CDOM浓度较高,太湖开敞区远离河口处,受外源河流的影响逐渐减小,且由于湖水的不断稀释,CDOM浓度不断降低.太湖东部水生植物很多,湖水较为清澈,CDOM浓度较低. |
关键词: 滇池 太湖 有色可溶性有机物(CDOM) 随机森林 哨兵-3A OLCI |
DOI:10.18307/2018.0411 |
分类号: |
基金项目:国家重点研发计划(2017YFB0503902)、国家自然科学基金项目(41471282)、国家水体污染控制与治理科技重大专项(2017ZX07302003)和民用航天技术预先研究项目联合资助. |
|
Estimation of CDOM concentration in inland lake based on random forest using Sentinel-3A OLCI |
WU Zhiming1, LI Jianchao1, WANG Rui1, SHI Lei1, MIAO Song1, LV Heng1,2, LI Yunmei1,2
|
1.Key Laboratory of Virtual Geographic Environment, Ministry of Education, Nanjing Normal University, Nanjing 210023, P. R. China;2.Jiangsu Center for Collaborative Innovation in Geographical Information Resource Development and Application, Nanjing 210023, P. R. China
|
Abstract: |
The chromophoric dissolved organic matter (CDOM) in the water is an important source of organic nutrients such as nitrogen and phosphorus in the lake ecosystem. It is a challenge to use the satellite data to inverse the CDOM concentration in inland water. Based on the in situ observations in Lake Dianchi (September 2009 and April 2017) and Lake Taihu (July 2016), and experiments in the laboratory and on the basis of analyzing the inherent optical properties of water bodies, this paper introduces a machine learning algorithm, the random forest inversion model of CDOM concentration in inland lake water, was established based on Sentinel-3A OLCI sensor. The inversion accuracy of the random forest model and other commonly used model such as band ratio model, the first order differential model, the semi-analysis model and the BP neural network model are evaluated by using an independent verification data set. The results show that the mean square error of the random forest model is 0.14 m-1, with a mean relative error 21%. Compared with the BP neural network model with relatively good inversion effect, the root mean square error is reduced by 50% and the mean relative error is reduced by 38%. The accuracy of inversion has been significantly improved. According to the characteristic parameters of the random forest algorithm, the influence of the respective variables is provided, it is found that the contribution rate of B11 (709 nm) and B6 (560 nm) is the largest, which is the sensitive band of CDOM. Finally, the random forest model was applied to the Sentinel-3A OLCI image in Lake Dianchi on April 12, 2017 and Lake Taihu on May 18, 2017, the distribution of CDOM concentration in the two lakes were obtained. The distribution of CDOM concentration in Lake Dianchi show that the northeast and the southwest region is higher than that in the central and western region. Meanwhile, the CDOM concentration in estuarine is higher than that in the lake water, which indicates that the input of runoff brings a lot of CDOM to Lake Dianchi. The distribution of CDOM concentration in Lake Taihu show a trend that higher in the west region while lower in the middle and east region. The western part of Lake Taihu and the Meiliang Bay in the northern part are greatly affected by the rivers inflow, resulted in a high CDOM concentration, although such influence is gradually reduced for the open area far from the estuary. The CDOM concentration in the eastern Lake Taihu is low due to the abundant aquatic plants and clear water. |
Key words: Lake Dianchi Lake Taihu chromophoric dissolved organic matter(CDOM) random forest Sentinel-3A OLCI |
|
|
|
|