引用本文: | 欧阳添,闪锟,周博天,黄昱,吴忠兴,尚明生.基于LSTM网络的在线藻类时序数据预测研究:以三峡水库为例.湖泊科学,2021,33(4):1031-1042. DOI:10.18307/2021.0406 |
| Ouyang Tian,Shan Kun,Zhou Botian,Huang Yu,Wu Zhongxing,Shang Mingsheng.Research on the online forecasting of algal kinetics based on time-series data and LSTM neural network:Taking Three Gorges Reservoir as an example. J. Lake Sci.2021,33(4):1031-1042. DOI:10.18307/2021.0406 |
|
本文已被:浏览 4098次 下载 1997次 |
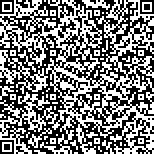 码上扫一扫! |
|
基于LSTM网络的在线藻类时序数据预测研究:以三峡水库为例 |
欧阳添1,2, 闪锟2, 周博天2, 黄昱2, 吴忠兴1, 尚明生2
|
1.西南大学三峡库区生态环境教育部重点实验室, 重庆市三峡库区植物生态与资源重点实验室, 重庆 400715;2.中国科学院重庆绿色智能技术研究院, 大数据与智能计算重庆市重点实验室, 重庆 400714
|
|
摘要: |
三峡水库在不同水位调控期支流回水区末端水深变化幅度较大,加之复杂水动力变化产生的生境异质性,塑造出有别于浅水湖泊的水华暴发特征.本研究基于库区4条支流——香溪河、澎溪河、大宁河及草堂河部署的自动监测数据,利用小波变换(WT)和长短期记忆网络(LSTM)构建藻类时序变化预测模型,并探讨神经网络层数、每层隐藏神经元数、时间步长数等关键参数的最优组合.结果表明:WT-LSTM模型可有效预测在线获取的叶绿素a浓度变化,模型在4条支流的均方根误差(RMSE)为0.049~0.221 μg/L,平均相对误差(MRE)为0.43%~1.12%;预测结果揭示深度神经网络方法可有效地提取在线藻类时序数据特征,而相较于深度置信网络(DBN),LSTM在4条支流叶绿素a预测的平均RMSE和MRE分别降低了9.20%和3.06%;在线监测数据的小波降噪并未影响叶绿素a的变化趋势,且WT-LSTM模型对叶绿素a预测效果显著提升于WT-DBN,平均RMSE和MRE分别降低了51.72%和59.24%;通过设置不同时间步长的预测实验,证实24 h内模型精度会随着预测步长的增加而降低,但模型平均相对误差可保持在13%以内,且对区间内叶绿素a极大值的预测精度要优于其平均值.本研究为水华预测上耦合在线监测与深度学习提供了研究范例,通过4个站点数据的交叉验证实验,亦证实具有统计学关联性的不同空间数据合并后可延展时序模型的学习样本,增强模型在实际应用中的稳健性. |
关键词: 在线监测 小波变换 长短期记忆网 浮游植物 三峡水库 |
DOI:10.18307/2021.0406 |
分类号: |
基金项目:重庆市技术创新与应用发展专项(cstc2019jscx-gksbX0042)、国家自然科学基金项目(51609229)、国家水体污染控制与治理科技重大专项(2014ZX07104-006)和中国科学院西部青年学者项目(E1296001)联合资助. |
|
Research on the online forecasting of algal kinetics based on time-series data and LSTM neural network:Taking Three Gorges Reservoir as an example |
Ouyang Tian1,2, Shan Kun2, Zhou Botian2, Huang Yu2, Wu Zhongxing1, Shang Mingsheng2
|
1.Key Laboratory of Eco-environments in Three Gorges Reservoir Region (Ministry of Education), Chongqing Key Laboratory of Plant Ecology and Resources Research in Three Gorges Reservoir Region, Southwest University, Chongqing 400715, P. R. China;2.Chongqing Institute of Green and Intelligent Technology, Chinese Academy of Sciences, Chongqing Key Laboratory of Big Data and Intelligent Computing, Chongqing 400714, P. R. China
|
Abstract: |
The water depth at the end of the tributary backwater area in the Three Gorges Reservoir (TGR) varies greatly, coupled with the habitat heterogeneity caused by complex hydrodynamic changes, shaping the characteristics of water bloom outbreaks that are different from shallow lakes. Based on the online monitoring system deployed in four tributaries of the TGR, this study uses wavelet transform (WT) and long short-term memory network (LSTM) to build the time-series forecasting model of algal kinetics, and discusses the optimal combination of key parameters such as the number of neural network layers, the number of hidden neurons in each layer, and the time steps. The results show that: WT-LSTM model can effectively predict the change of chlorophyll-a concentrations in four tributaries, the root mean square error (RMSE) is 0.049-0.221 μg/L, and the mean relative error (MRE) is 0.43%-1.12%. This study confirms that the deep learning model can learn inherent patterns of high-frequency monitoring data, and the mean RMSE and MRE in the four tributaries are decreased by 9.20% and 3.06%, respectively. After online data processing with the wavelet transform, the prediction performance of WT-LSTM is also better than WT-DBN, and the mean RMSE and MRE decreased by 51.72% and 59.24%, respectively. Comparison experiments with different time steps confirm that the accuracy of the model decreases with the improvement of the prediction time. While the mean relative error of the prediction task within 24 hours is less than 13%, and the prediction ability of the model to chlorophyll-a concentration of the interval maximum is better than the average. This study provides a research example for the combination of automatic monitoring data and deep neural network models to forecast harmful algal blooms. Through cross-validation experiments on data from four sites, it is confirmed that statistically relevant data can be extended for model training and testing samples, enhancing the stability of machine learning models in practical applications. |
Key words: On-line monitoring wavelet transform long short-term memory phytoplankton Three Gorges Reservoir |