引用本文: | 汪志成,王杰,晏实江,崔玉环,王行行.基于Sentinel-3 OLCI影像的巢湖藻蓝素浓度年内动态遥感监测.湖泊科学,2022,34(2):391-403. DOI:10.18307/2022.0203 |
| Wang Zhicheng,Wang Jie,Yan Shijiang,Cui Yuhuan,Wang Hanghang.Annual dynamic remote sensing monitoring of phycocyanin concentration in Lake Chaohu based on Sentinel-3 OLCI images. J. Lake Sci.2022,34(2):391-403. DOI:10.18307/2022.0203 |
|
本文已被:浏览 3509次 下载 2642次 |
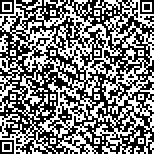 码上扫一扫! |
|
基于Sentinel-3 OLCI影像的巢湖藻蓝素浓度年内动态遥感监测 |
汪志成1,2, 王杰1,2,3, 晏实江1,3, 崔玉环4, 王行行1
|
1.安徽大学资源与环境工程学院, 合肥 230601;2.安徽大学湿地生态保护与修复安徽省重点实验室, 合肥 230601;3.安徽省地理信息工程中心, 合肥 230601;4.安徽农业大学理学院, 合肥 230036
|
|
摘要: |
藻蓝素(PC)是水体蓝藻的指示性色素,其浓度反映了蓝藻生物量,利用卫星遥感监测藻蓝素浓度年内动态对蓝藻水华的有效防控有着重要意义.根据不同季节的巢湖藻蓝素浓度实测数据与同期Sentinel-3 OLCI影像,构建机器学习回归反演模型,应用于巢湖2019年OLCI影像集上,对巢湖藻蓝素浓度的空间分布、年内变化进行遥感监测.研究表明:在MUMM和C2RCC水体大气校正方法中,C2RCC的大气校正结果更接近实测光谱反射率;在机器学习回归算法中,基于梯度提升回归的藻蓝素浓度反演模型精度最高,其R2、RMSE和rRMSE分别达到0.84、49.76 μg/L和34.1%.水体藻蓝素浓度在1—4月及12月较低,在5—11月浓度较高且波动频繁,日均气温是水体藻蓝素浓度年内变化的主要原因,而藻蓝素短期剧烈波动主要是受到日降水量和日照时数的影响;在夏、秋季节,西湖区藻蓝素浓度明显高于中、东湖区,主要与入湖河流中氮磷等物质的高输入有关.Sentinel-3 OLCI影像为湖库水体藻蓝素浓度动态监测提供了重要数据源,梯度提升回归算法在富营养化水体藻蓝素浓度反演中具有较大的应用潜力. |
关键词: Sentinel-3 OLCI 机器学习 藻蓝素 遥感反演 时空变化 巢湖 |
DOI:10.18307/2022.0203 |
分类号: |
基金项目:国家自然科学基金项目(32171573,41401022)、安徽省科技重大专项(201903a07020014)和安徽高校自然科学研究项目(KJ2019A0045)联合资助. |
|
Annual dynamic remote sensing monitoring of phycocyanin concentration in Lake Chaohu based on Sentinel-3 OLCI images |
Wang Zhicheng1,2, Wang Jie1,2,3, Yan Shijiang1,3, Cui Yuhuan4, Wang Hanghang1
|
1.School of Resources and Environmental Engineering, Anhui University, Hefei 230601, P. R. China;2.Anhui Province Key Laboratory of Wetland Ecosystem Protection and Restoration, Anhui University, Hefei 230601, P. R. China;3.Engineering Center for Geographic Information of Anhui Province, Hefei 230601, P. R. China;4.School of Science, Anhui Agricultural University, Hefei 230036, P. R. China
|
Abstract: |
Phycocyanin (PC) is an indicator pigment of cyanobacteria in water, and its concentration reflects the biomass of cyanobacteria. Monitoring the annual dynamics of PC concentration by satellite remote sensing is of great significance for the effective prevention and control of cyanobacteria bloom. Based on the measured data of phycocyanin concentration in Lake Chaohu in different seasons and the Sentinel-3 OLCI images of the same period, a machine learning regression retrieval model was constructed and applied to the 2019 OLCI image set of Lake Chaohu to monitor the spatial distribution and annual variation of PC concentration. The results show that among MUMM and C2RCC atmospheric correction methods, the atmospheric correction result of C2RCC is closer to the measured spectral reflectance. Among the machine learning regression algorithms, the retrieval model of PC concentration based on gradient boosting regression has the highest accuracy, its R2, RMSE and rRMSE reach 0.84, 49.76 μg/L and 34.1%, respectively. The concentration of PC was low from January to April and December, but high from May to November and fluctuated frequently. The average daily temperature was the main reason for the annual variation of PC concentration, and the short-term fluctuation of PC was mainly affected by the daily precipitation and sunshine duration. In summer and autumn, the PC concentration in the West Lake area was significantly higher than that in the middle and east lake area, which was mainly related to the high input of nitrogen and phosphorus into the lake. Sentinel-3 OLCI images provide important data sources for the dynamic monitoring of PC concentration in lake and reservoir waters, and the gradient boosting regression algorithm has great application potential in the retrieval of PC concentration in eutrophication waters. |
Key words: Sentinel-3 OLCI machine learning phycocyanin remote sensing retrieval spatiotemporal variation Lake Chaohu |