附件
引用本文: | 何孟奇,闪锟,王兰,杨柳,李哲,尚明生.基于非线性时序分析的三峡支流浮游植物因果响应.湖泊科学,2023,35(2):507-518. DOI:10.18307/2023.0209 |
| He Mengqi,Shan Kun,Wang Lan,Yang Liu,Li Zhe,Shang Mingsheng.Nonlinear time-series analysis on the causality response of phytoplankton in a tributary of the Three Gorges Reservoir. J. Lake Sci.2023,35(2):507-518. DOI:10.18307/2023.0209 |
|
|
|
本文已被:浏览 2585次 下载 2845次 |
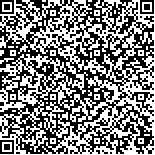 码上扫一扫! |
|
基于非线性时序分析的三峡支流浮游植物因果响应 |
何孟奇1,2, 闪锟2, 王兰3,4, 杨柳2, 李哲2, 尚明生2
|
1.西华师范大学计算机学院, 南充 637009;2.中国科学院重庆绿色智能技术研究院, 中国科学院水库水环境重点实验室, 重庆 400714;3.重庆邮电大学计算机科学与技术学院, 重庆 400065;4.重庆第二师范学院人工智能学院, 重庆 400065
|
|
摘要: |
水华的频发已成为当前三峡水库最为突出的生态环境问题之一。尽管水动力调控叠加上温度变暖和营养负荷增加会诱导水华暴发强度和频率增加,但仍缺乏有效的方法框架去利用野外观测数据评估环境因子与浮游植物间的因果关联。本研究以三峡水库澎溪河监测数据为例,采用非线性时序分析的建模框架来量化浮游植物的因果响应规律。数据来自于2007年6月至2018年9月澎溪河流域的高阳平湖和汉丰湖两个观测点,其中包含了水文、气象和水质及叶绿素a等11种变量。首先,利用奇异谱分析(SSA)分离了叶绿素a和环境因子的低维确定性动力学信号;其次,采用收敛交叉映射(CCM)方法检验了叶绿素a与环境因子间的因果关联。结果显示:① 气象因子、支流流量、水温、三峡大坝水位和上游调节坝水位是影响高阳平湖叶绿素a时序变化的重要因素;② 总氮、总磷为代表的营养盐只在汉丰湖观测点中表现出与叶绿素a的因果关系,且总氮较之于总磷对叶绿素a变化影响更为显著;③ CCM结果与传统的皮尔森相关性分析及格兰杰因果检验比较,证实非线性时序分析方法在分析浮游植物的因果响应上更具优势。本研究为水生态系统的因果建模提供了研究范例,也为推动利用长期观测数据评估三峡水库水华驱动因子提供了新的研究角度。 |
关键词: 三峡水库 澎溪河 有害水华 环境因子 水位调控 因果分析 非线性时序分析 |
DOI:10.18307/2023.0209 |
分类号: |
基金项目:国家自然科学基金项目(62072429,51609229)、中国科学院西部之光基金项目(E1296001)、重庆市教委重点合作项目(HZ2021008)、云南省市一体化重点科技项目(202202AH210006)、重庆市技术创新与应用发展专项重点项目(CSTB2022TIAD-KPX0199)和国家重点研发计划项目(2022YFC3203504)联合资助。 |
|
Nonlinear time-series analysis on the causality response of phytoplankton in a tributary of the Three Gorges Reservoir |
He Mengqi1,2, Shan Kun2, Wang Lan3,4, Yang Liu2, Li Zhe2, Shang Mingsheng2
|
1.School of Computer Science, China West Normal University, Nanchong 637009, P.R.China;2.Chongqing Institute of Green and Intelligent Technology, Chinese Academy of Sciences, CAS Key Lab on Reservoir Environment, Chongqing 400714, P. R. China;3.College of Computer Science and Technology, Chongqing University of Posts and Telecommunications, Chongqing 400065, P. R. China;4.School of Artificial Intelligence, Chongqing University of Education, Chongqing 400065, P. R. China
|
Abstract: |
Harmful algae blooms in Three Gorges Reservoir (TGR) have become the most major environmental and ecological problem. Despite broad recognition that hydrological manipulation, warming climate and nutrient loading promote the intensity and frequency of bloom events, the main problem is still no science-based framework for evaluating the causal relationships between environmental factors and phytoplankton biomass by using field observation data. Thus, as a case study, a nonlinear time-series causality analysis framework was used to reveal the phytoplankton response in the TGR, China. Two sampling stations, named Lake Gaoyangping and Lake Hanfeng, were used to reconstruct the dynamics of real-world environmental systems with eleven parameters from June 2007 to September 2018. Singular spectrum analysis isolated low-dimensional, deterministic signals for chlorophyll-a(Chl.a) dynamics and other candidate drivers. Causal analysis with convergent cross-mapping (CCM) provided strong evidence that temperature, sunshine hours, precipitation, discharge in the tributary, and water level of TGR systematically influenced phytoplankton biomass dynamics in Lake Gaoyangping. In contrast, total nitrogen (TN) and total phosphorus (TP) showed a causal relationship with Chl.a only in Lake Hanfeng, and the effect of TN on Chl.a was more significant than that of TP. Finally, the research compares the nonlinear CCM analysis with the linear Pearson correlation analysis and Granger causality test. It confirms that the nonlinear causality method has more advantage in causal discovery based on long time-series monitoring data. Overall, this study provides a case for causal modelling of water ecosystems and a new research perspective for evaluating the environmental drivers of harmful algae blooms in TGR by using long-term observational data. |
Key words: Three Gorges Reservoir Pengxi River harmful algal blooms environmental factors water-level manipulation causality analysis nonlinear time-series analysis |
|
|
|
|