附件
引用本文: | 胡乐怡,蒋晓蕾,周嘉慧,欧阳芬,戴逸姝,章丽萍,付晓雷.考虑记忆时间的LSTM模型在赣江流域径流预报中的应用.湖泊科学,2024,36(4):1241-1251. DOI:10.18307/2024.0454 |
| Hu Leyi,Jiang Xiaolei,Zhou Jiahui,Ouyang Fen,Dai Yishu,Zhang Liping,Fu Xiaolei.Application of LSTM considering time steps in runoff prediction of Ganjiang River Basin. J. Lake Sci.2024,36(4):1241-1251. DOI:10.18307/2024.0454 |
|
|
|
本文已被:浏览 2738次 下载 2023次 |
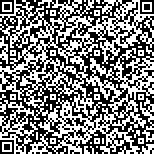 码上扫一扫! |
|
考虑记忆时间的LSTM模型在赣江流域径流预报中的应用 |
胡乐怡1, 蒋晓蕾1,2,3, 周嘉慧1, 欧阳芬4, 戴逸姝1, 章丽萍1, 付晓雷1,2,3
|
1.扬州大学水利科学与工程学院, 扬州 225009;2.南京信息工程大学水利部水文气象灾害机理与预警重点实验室, 南京 210044;3.河海大学水灾害防御全国重点实验室, 南京 210098;4.南昌工程学院水利与生态工程学院, 南昌 330099
|
|
摘要: |
在气候变化条件下,准确的径流预测对水资源的规划与管理十分重要。本文基于长短时记忆神经网络(LSTM)模型,采用赣江流域外洲、峡江以及栋背水文站的逐日流量以及CN05.1日降水数据构建3个不同面积流域的径流预测模型,并通过设置不同情景分析:模型的有效预见期与不同流域平均产汇流时间之间的关系,有效预见期内LSTM径流预测模型精度与记忆时间之间的关系,不同长度的预见期与模型最佳记忆时间之间的关系,同时探讨LSTM径流预测所需的记忆时间与流域面积的关系。结果表明:(1)综合考虑降水和前期径流情景下的径流预测效果最好,当预见期为1 d时,外洲、峡江、栋背站的纳什效率系数(NSE)分别可达0.98、0.96以及0.90;且其有效预见期与仅考虑降水信息的有效预见期相同,均与流域平均产汇流时间相近。(2)随着预见期的延长,不同情景下的预测精度均有不同程度的下降,其中仅考虑前期径流情景的下降率最大,说明降水信息较前期径流对径流预测效果的提升更重要。同时,随着流域面积的增加,相同预见期内径流预测精度均有所提升。(3)当预见期相同时,随记忆时间的延长,不同径流预测模型的预测精度均先上升至最高,接着具有下降趋势,最后逐渐趋于稳定。且在有效预见期内,随着预见期的延长,最佳记忆时间均有增大趋势,当达到最长的有效预见期时,对应的最佳记忆时间均为14 d。此外,在赣江流域的模拟结果表明,随着流域面积的增大,LSTM的最佳记忆时间减小。研究结果可为赣江流域的径流预报提供参考,同时有助于推求其他流域采用机器学习进行径流预测所需的最佳记忆时间。 |
关键词: LSTM模型 赣江流域 记忆时间 径流预测 预见期 |
DOI:10.18307/2024.0454 |
分类号: |
基金项目:国家自然科学基金项目(42371021, 52109036)、河海大学水灾害防御全国重点实验室“一带一路”水与可持续发展科技基金面上项目(2022491111, 2021490611)、水利部水文气象灾害机理与预警重点实验室开放基金(HYMED202203, HYMED202210)和江苏省研究生科研与实践创新计划项目(KYCX23_3546, KYCX23_3549)联合资助。 |
|
Application of LSTM considering time steps in runoff prediction of Ganjiang River Basin |
Hu Leyi1, Jiang Xiaolei1,2,3, Zhou Jiahui1, Ouyang Fen4, Dai Yishu1, Zhang Liping1, Fu Xiaolei1,2,3
|
1.College of Hydraulic Science and Engineering, Yangzhou University, Yangzhou 225009, P. R. China;2.Key Laboratory of Hydrometeorological Disaster Mechanism and Warning of Ministry of Water Resources, Nanjing University of Information Science & Technology, Nanjing 210044, P. R. China;3.The National Key Laboratory of Water Disaster Prevention, Hohai University, Nanjing 210098, P. R. China;4.School of Hydraulic and Ecological Engineering, Nanchang Institute of Technology, Nanchang 330099, P. R. China
|
Abstract: |
Accurate forecast of runoff is important for water resources management and planning under climate change. This study developed a Long Short-Term Memory (LSTM) neural network model for runoff forecasting at three catchments (Waizhou, Xiajiang, and Dongbei) in Ganjiang River Basin. Based on the developed LSTM, we analyzed the relationship between the effective lead time of the model and watershed average transit time of different basins, between the accuracy of the LSTM runoff prediction model and time steps during the effective lead time, between different lead times and the best time steps of the model, and the relationship between the time steps required for LSTM runoff prediction and the watershed area. The results showed that (1) the best model fit can be achieved by considering both precipitation and antecedent runoff. The Nash-Sutcliffe efficiency coefficient (NSE) for the Waizhou, Xiajiang and Dongbei stations reached 0.98, 0.96 and 0.90, respectively under 1-day lead time. Moreover, the effective lead time is the same as the one which only considering the information of precipitation, and both are close to the watershed average transit time. (2) By extending the lead time, the model fit decreased under different scenarios, with the largest decreasing rate from the scenario that only considered the antecedent runoff. This indicated that precipitation was more important for runoff prediction. Additionally, the accuracy of runoff prediction increased with larger watershed area. (3) By fixing the lead time, the model fit firstly improved, then decreased to gradually stabilize with the extension of the time steps. During the effective lead time, the best time steps increased when extending the lead time, and the best time steps were 14 days for the longest effective lead time. The best time steps of LSTM decreased with a larger catchment area in the Ganjiang River Basin. The results can be taken as a reference for runoff forecasting in Ganjiang River Basin, and are helpful for obtaining the best time steps of machine learning or runoff prediction in other basins. |
Key words: LSTM model Ganjiang River Basin time steps runoff prediction lead time |
|
|
|
|